Outline of an HPSG-style Chinese reversible grammar*
Wei LI
Simon Fraser University
(NLWC97)
This paper presents the outline and the design philosophy of a lexicalized Chinese unification grammar named W‑CPSG. W‑CPSG covers Chinese morphology, Chinese syntax and semantics in a novel integrated language model. The grammar works reversibly, suited for both parsing and generation. This work is developed in the general spirit of the linguistic theory Head-driven Phrase Structure Grammar (Pollard & Sag 1994). We identify the following two problems as major obstacles in formulating a precise and efficient Chinese grammar. First, we lack in serious study on Chinese lexical base and often jump too soon for linguistic generalization. Second, there is a lack of effective interaction and adequate interface between morphology, syntax and semantics. We address these problems in depth with the lexicalized and integrated design of W‑CPSG. We will also illustrate how W‑CPSG is formalized and how it works.
-
Background
Unification grammars have been extensively studied in the last decade (Shieber 1986). Implementations of such grammars for English are being used in a wide variety of applications. Attempts also have been made to write Chinese unification grammars (Huang 1986, among others). W‑CPSG (for Wei's Chinese Phrase Structure Grammar, Li, W. 1997b) is a new endeavor in this direction, with its unique design and characteristics.
1.1. Design philosophy
We identify the following two problems as major obstacles in formulating a precise and efficient Chinese grammar. First, we lack in serious study on Chinese lexical base and often jump too soon for linguistic generalization. Second, there is a lack of effective interaction and adequate interface between morphology, syntax and semantics. We address these problems in depth with the lexicalized and integrated design of W‑CPSG.
1.1.1. Lexicalized design
It has been widely accepted that a well-designed lexicon is crucial for a successful grammar, especially for a natural language computational system. But Chinese linguistics in general and Chinese computational grammars in particular have generally been lacking in in-depth research on Chinese lexical base. For many years, most dictionaries published in China did not even contain information for grammatical categories in the lexical entries (except for a few dictionaries intended for foreign readers learning Chinese). Compared with the sophisticated design and rich linguistic information embodied in English dictionaries like Oxford Advanced Learners' Dictionary and Longman Dictionary of Contemporary English, Chinese linguistics is hampered by the lack of such reliable lexical resources.
In the last decade, however, Chinese linguists have achieved significant progress in this field. The publication of 800 Words in Contemporary Mandarin (Lü et al., 1980) marked a milestone for Chinese lexical research. This book is full of detailed linguistic description of the most frequently used Chinese words and their collocations. Since then, Chinese linguists have made fruitful efforts, marked by the publication of a series of valency dictionaries (e.g. Meng et al., 1987) and books (e.g. Li, L. 1986, 1990). But almost all such work was done by linguists with little knowledge of computational linguistics. Their description lacks formalization and consistency. Therefore, Chinese computational linguists require patience in adapting and formalizing these results, making them implementable.
1.1.2. Integrated design
Most conventional grammars assume a successive model of morphology, syntax and semantics. We argue that this design is not adequate for Chinese natural language processing. Instead, an integrated grammar of morphology, syntax and semantics is adopted in W‑CPSG.
Let us first discuss the rationale of integrating morphology and syntax in Chinese grammar. As it stands, a written Chinese sentence is a string of characters (morphemes) with no blanks to mark word boundaries. In conventional systems, there is a procedure-based Chinese morphology preprocessor (so-called segmenter). The major purpose for the segmenter is to identify a string of words to feed syntax. This is not an easy task, due to the possible involvement of the segmentation ambiguity. For example, given a string of 4 Chinese characters da xue sheng huo, the segmentation ambiguity is shown in (1a) and (1b) below.
(1) da xue sheng huo
(a) da-xue | sheng-huo
university | life
(b) da-xue-sheng | huo
university-student | live
The resolution of the above ambiguity in the morphology preprocessor is a hopeless job because such structural ambiguity is syntactically conditioned. For sentences like da xue sheng huo you qu (university life is interesting), (1a) is the right identification. For sentences like da xue sheng huo bu xia qu le (university students cannot make a living), (1b) is right. So far there are no segmenters which can handle this properly and guarantee correct word segmentation (Feng 1996). In fact, there can never be such segmenters as long as syntax is not brought in. This is a theoretical defect of all Chinese analysis systems in the morphology-before-syntax architecture (Li, W. 1997a). I have solved this problem in our morphology-syntax integrated W‑CPSG (see 2.2. below).
Now we examine the motivation of integrating syntax and semantics in Chinese grammar. It has been observed that, compared with the analysis of Indo-European languages, proper Chinese analysis relies more heavily on semantic information (see, e.g. Chen 1996, Feng 1996). Chinese syntax is not as rigid as languages with inflections. Semantic constraint is called for in both structural and lexical disambiguation as well as in solving the problem of computational complexity. The integration of syntax and semantics helps establish flexible ways of their interaction in analysis (see 2.3. below).
1.2. Major theoretical foundation: HPSG
The work on W‑CPSG is developed in the spirit of the linguistic theory Head-driven Phrase Structure Grammar (HPSG, proposed by Pollard & Sag, 1987). HPSG is a highly lexicalist theory, which encourages the integration of different components. This matches our design philosophy for implementing our Chinese computational grammar. HPSG serves as a desired framework to start this research with. We benefit most from the general linguistic ideas in HPSG. However, W‑CPSG is not confined to the theory-internal formulations of principles and rules and other details in HPSG versions (e.g. Pollard & Sag 1987, 1994 or later developments). We borrow freely from other theoretical sources or form our own theories in W‑CPSG to meet our goal of Natural Language Processing in general and Chinese computing in particular. For example, treating morphology as an integrated part of parsing and placing it right into grammar is our deliberate choice. In syntax, we formulate our own theory for configuration and word order. Our semantics differs most from any standard version of situation-semantics-based theory in HPSG. It is based on insights from Tesnière's Dependency Grammar (Tesnière 1959), Fillmore's Case Grammar (Fillmore 1968) and Wilks' Preference Semantics (Wilks 1975, 1978) as well as our own semantic view for knowledge representation and better coordination of syntax-semantics interaction (Li, W. 1996). For these differences and other modifications, it is more accurate to regard W‑CPSG as an HPSG-style Chinese grammar, rather than an (adapted) version of Chinese HPSG.
-
Integrated language model
2.1. W‑CPSG versus conventional Chinese grammar
The lexicalized design sets the common basis for the organization of the grammar in W‑CPSG. This involves the interfaces of morphology, syntax and semantics.[1] W‑CPSG assumes an integrated language model of its components (see Figure 1). The W‑CPSG model is in sharp contrast to the conventional clear-cut successive design of grammar components (see Figure 2).
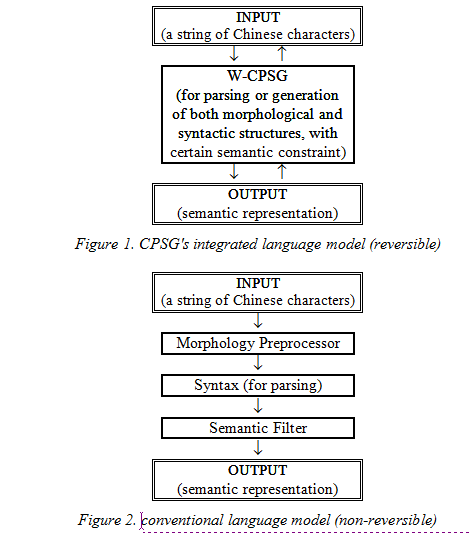
Figure 2. conventional language model (non-reversible)
2.2. Interfacing morphology and syntax
As shown in Figure 2 above, conventional systems take a two-step approach: a procedure-based preprocessor for word identification (without discovering the internal structure) and a grammar for word-based parsing. W‑CPSG takes an alternative one-step approach and the parsing is character- (i.e. morpheme-) based. A morphological PS (phrase structure) rule is designed not only to identify candidate words but to build word‑internal structures as well. In other words, W‑CPSG is a self-contained model, directly accepting the input of a character string for parsing. The parse tree embodies both the morphological analysis and the syntactic analysis, as illustrated by the following sample parsing chart.
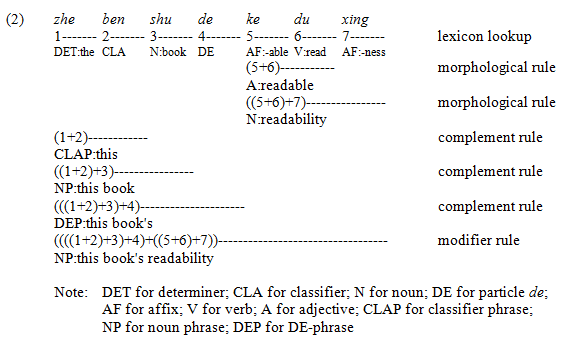
Note: DET for determiner; CLA for classifier; N for noun; DE for particle de;
AF for affix; V for verb; A for adjective; CLAP for classifier phrase;
NP for noun phrase; DEP for DE-phrase
This is so-called bottom-up parsing. It starts with lexicon look-up. Simple edges 1 through 7 are lexical edges. Combined edges are phrasal edges. Each edge represents a sign, i.e. a character (morpheme), a word, a phrase or a sentence. Lexical edges result from a successful match between the signs in the input string and the entries in the lexicon during lexicon look-up. After looking up the lexicon, the lexical information for the signs are made available to the parser. For the sake of concise illustration, we only show two crucial pieces of information for each edge in the chart, namely category and interpretation with a delimiting colon (some function words are only labeled for category). The parser attempts to combine the edges according to PS rules in the grammar until a parse is found. A parse is an edge which ranges over the whole string. The parse ((((1+2)+3)+4)+((5+6)+7)) represents the following binary structural tree embodying both the morphological and syntactic analysis of this NP phrase.
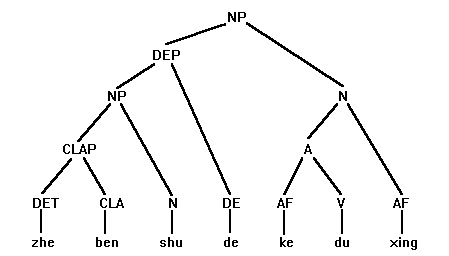
As seen, word identification is no longer a pre-condition for parsing. It becomes a natural by-product of parsing in this integrated grammar of morphology and syntax: a successful parse always embodies the right word identification. For example, the parse ((((1+2)+3)+4)+((5+6)+7)) includes the identification of a word-string zhe (DET) ben (CLA) shu (N) de (DE) ke-du-xing (N). An argument against the conventional separation model is that there exists in the two-step approach a theoretical threshold beyond which the precision for the correct word identification is not possible. This is because proper word identification in Chinese is to a considerable extent syntactically conditioned due to possible structural ambiguity involved. Our strategy has advantages over the conventional approach in resolving word identification ambiguities and in handling the productive word formation. It has solved the problems inherent in the morphology-before-syntax architecture (for detailed argumentation, see Li, W. 1997a).
2.3. Interaction of syntax and semantics
The interface and interaction of syntax and semantics are of vital importance in a Chinese grammar. We are of the same opinion as Chen (1996) and many others that it is more effective to analyze Chinese in an environment where semantic constraints are enforced during the parsing, not after. The argument is based on the linguistic characteristics of Chinese. Chinese has no inflection (like English ‑'s, ‑s, ‑ing, ‑ed, etc.), no such formatives as article (like English a, the), infinitivizer (like English to) and complementizer (like English that). Instead, function words and word order are used as major syntactic devices. But Chinese function words (prepositions, aspect particles, passive particle, plural suffix, conjunctions, etc.) can often be omitted (Lü et al. 1980, p.2). Moreover, fixed word order in order to mark syntactic functions which is usually assumed for isolating languages, is to a considerable extent untrue for Chinese. In fact, there is remarkable freedom or flexibility in Chinese word order. One typical example is demonstrated in the numerous word order variations (although the default order is S‑V‑O subject-verb-object) for the Chinese transitive patterns (Li, W. 1996). All these added up project a picture of Chinese as a language of loose syntactic constraint. A weak syntax requires some support beyond syntax to enhance grammaticality. Semantic constraints are therefore called for. I believe that an effective way to model this interaction between syntax and semantics is to integrate the two in one grammar.
One strong piece of evidence for this syntax-semantics integration argument is that Chinese has what I call syntactically crippled structures. These are structures which can hardly be understood on purely formal grounds and are usually judged as ungrammatical unless accompanied with the support from the semantic constraints (i.e. the match of semantic selection restrictions). Some Chinese NP predicate (Li, W. & McFetridge 1995) and transitive patterns like S‑O‑V (Li, W. 1996), among others, are such structures. The NP Predicate is a typical instance of semantic dependence. It is highly undesirable if we assume a general rule like S --> NP1 NP2 in a Chinese grammar to capture such phenomena. This is because there is a semantic condition for NP2 to function as predicate, which makes the Chinese NP predicate a very restricted pattern. For example, in the sentence This table is three-legged: zhe (this) zhang (classifier) zhuo-zi (desk) san (three) tiao (classifier) tui (leg), the subject must be of the semantic type animate or furniture (which can have legs). The general rule with no recourse to semantic constraints is simply too productive and may cause severe computational complexity. In the case of Chinese transitive patterns, formal means are decisive for some variations in their interpretation (i.e. role assignment) process. But others are heavily dependent on semantic constraint. Take chi (eat) as an example. There is no difference in syntactic form in sentences like wo (I) chi (eat) dianxin (Dim-Sum) le (perfect-aspect) and dianxin (Dim-Sum) wo (I) chi (eat) le (perfect-aspect). Who eats what? To properly assign roles to NP1 NP2 V as S-O-V versus O-S-V, the semantic constraint animate eats food needs to be enforced.
The conventional syntax-before-semantics model has now received less popularity in Chinese computing community. Researchers have been exploring various ways of integrating syntax and semantics in Chinese grammar (Chen 1996). In W‑CPSG, the Chinese syntax was enhanced by the incorporation of a semantic constraint mechanism. This mechanism embodies a lexicalized knowledge representation, which parallels to the syntactic representation in the lexicon. I have developed a way to dynamically coordinate the syntactic constraint and semantic constraint in one model. This technique proves to be effective in handling rhetorical expressions and in making the grammar both precise and robust (Li, W 1996).
-
Lexicalized formal grammar
3.1. Formalized grammar
The application nature of this research requires that we pay equal attention to practical issues of computational systems as well as to a sound theoretical design. All theories and rule formulations in W‑CPSG are implementable. In fact. most of them have been implemented in our prototype W‑CPSG. W‑CPSG is a strictly formalized grammar that does not rely on undefined notions. The whole grammar is represented by typed feature structures (TFS), as defined below based on Carpenter & Penn (1994).
(3) Definition: typed feature structure
A typed feature structure is a data structure adopted to model a certain object of a grammar. The necessary part for a typed feature structure is type. Type represents the classification of the feature structure. A simple feature structure contains only the type information, but a complex feature structure can introduce a set of feature-value pairs in addition to the type. A feature-value pair consists of a feature and a value. A feature reflects one aspect of an object. The value describes that aspect. A value is itself a feature structure (simple or complex). A feature determines which type of feature structures it takes as its value. Typed feature structures are finite in a grammar. Their definition constitutes the typology of the grammar.
With this formal device of typed feature structures, we formulate W‑CPSG by defining from the very basic notions (e.g. sign, morpheme, word, phrase, S, NP, VP, etc.) to rules (PS rules and lexical rules), lexical items, lexical hierarchy and typology (hierarchy embodied in feature structures) (Li, W. 1997b). The following sample definitions of some basic notions illustrate the formal nature of W‑CPSG. Please note that they are system-internal definitions and are used in W‑CPSG to serve the purpose of configurational constraints (see Chapter VI of Li, W. 1997b).
(4) Definition: sign [2]
a_sign
KANJI kanji
MORPH expected
CATEGORY category
COMP0 expected
COMP1 expected
COMP2 expected
MOD expected
KNOWLEDGE knowledge
CONTENT content
DTR dtr
A sign is the most fundamental concept of grammar. A sign is a dynamic unit of grammatical analysis. It can be a morpheme, a word, a phrase or a sentence. Formally, a sign is defined by the TFS a_sign, which introduces a set of linguistic features for its description, as shown above. These features include the orthographic feature KANJI; morphological feature MORPH; syntactic features CATEGORY, COMP0, COMP1, COMP2, and MOD; structural feature (for both morphology and syntax) DTR; semantic features KNOWLEDGE and CONTENT.
(5) Definition: morpheme
a_sign
MORPH ~saturated
A morpheme is a sign whose morphological expectation has not been saturated. In W‑CPSG, ~saturated is equivalent to obligatory/optional/null. For example, the suffix ‑xing (‑ness) is such a morpheme whose morphological expectation for a preceding adjective is obligatory. In W‑CPSG, a morpheme like ‑xing (‑ness) ceases to be a morpheme when its obligatory expectation, say the adjective ke-du (readable), is saturated. Therefore, the sign ke-du-xing (readability) is not a morpheme, but becomes a word per se.
(6) Definition: word
a_sign
MORPH ~obligatory
DTR no_syn_dtr
In W‑CPSG, ~obligatory is equivalent to saturated/optional/null. The specification [MORPH ~obligatory] defines a syntactic sign, i.e. a sign whose obligatory morphological expectation has been saturated. A word is a syntactic sign with no syntactic daughters, i.e. [DTR no_syn_dtr]. Obviously, word with [MORPH saturated/optional/null] overlaps morpheme with [MORPH obligatory/optional/null] in cases when the morphological expectation is optional or null.
Just like the overlapping of morpheme and word, there is also an intersection between word and phrase. Compare the following definition of phrase with the above definition of word.
(7) Definition: phrase
a_sign
MORPH ~obligatory
COMP0 ~obligatory
COMP1 ~obligatory
COMP2 ~obligatory
A phrase is a syntactic sign whose obligatory complement expectation has all been saturated, i.e. [COMP0 ~obligatory, COMP1 ~obligatory, COMP2 ~obligatory]. When a word has only optional complement expectation or no complement expectation, it is also a phrase. The overlapping relationship among morpheme, word and phrase can be shown by the following illustration of the three sets.
S is a syntactic sign satisfying the following 3 conditions: (1) its category is pred (which includes V and A); (2) its comp0 is saturated; (3) its obligatory comp1 and comp2 are saturated.
3.2. Lexicalized grammar
W‑CPSG takes a radical lexicalist approach. We started with individual words in the lexicon and have gradually built up a lexical hierarchy and the grammar prototype.
W‑CPSG consists of two parts: a minimized general grammar and a information-enriched lexicon. The general grammar contains only 11 PS rules, covering complement structure, modifier structure, conjunctive structure and morphological structure. We formulate a PS rule for illustration.
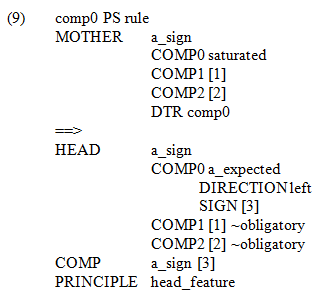
This comp0 PS rule is similar to the rule S ==> NP VP in the conventional phrase structure grammar. The feature COMP0 represents the expectation of the head daughter for its external complement (subject or specifier) on its left side, i.e. [DIRECTION left]. The nature of its expected comp0, NP or other types of sign, is lexically decided by the individual head (hence head-driven or lexicon-driven). It will always be warranted by the general grammar, here via the index [3]. This is the nature of lexicalized grammars. PS rules in such grammars are very abstract. Essentially, they say one thing, namely, 2 signs can combine so long as the lexicon so indicates. The indices [1] and [2] represent configurational constraint. They ensure that internal obligatory complements COMP1 and COMP2 must be saturated before this rule can be applied. Finally, Head Feature Principle (defined elsewhere in the grammar based on the adaptation of the Head Feature Principle in HPSG, Pollard & Sag, 1994) ensures that head features are percolated up from the head daughter to the mother sign.
The lexicon houses lexical entries with their linguistic description and knowledge representation. Potential morphological structures, as well as potential syntactic structures, are lexically encoded (in the feature MORPH for the former and in the features COMP0, COMP1, COMP2, MOD for the latter). Our knowledge representation is also embodied in the lexicon (in the feature KNOWLEDGE). I believe that this is an effective and realistic way of handling natural language phenomena and their disambiguation without having to resort to an encyclopedia-like knowledge base. The following sample formulation of the lexical entry chi (eat) projects a rough picture of what the W‑CPSG lexicon looks like.
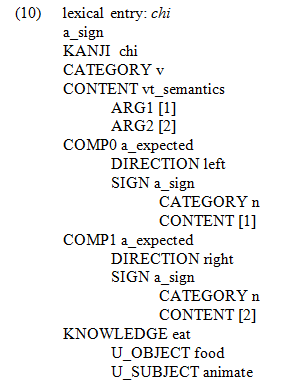
The lexicon also contains lexical generalizations. The generalizations are captured by the inheritance of the lexical hierarchy and by a set of lexical rules. Due to space limitations, I will not show them in this paper.
-
Implementation and application of W‑CPSG
A substantial Chinese computational grammar has been implemented in the W‑CPSG prototype. It covers all basic Chinese constructions. Particular attention is paid to the handling of function words and verb patterns. On the basis of the information- enriched lexicon and the general grammar, the system adequately handles the relationship between linguistic individuality and generality. The grammar formalism which I use to code W‑CPSG is ALE, a grammar compiler on top of Prolog, developed by Carpenter & Penn (1994). ALE is equipped with an inheritance mechanism on typed feature structures, a powerful tool in grammar modeling. I have made extensive use of the mechanism in the description of lexical categories as well as in knowledge representation. This seems to be an adequate way of capturing the inherent relationship between features in a grammar. Prolog is a programming environment particularly suitable for the development of unification and reversible grammars (Huang 1986, 1987). ALE compiles W‑CPSG into a Chinese parser, a Prolog program ready to accept a string of characters for analysis. In the first experiment, W‑CPSG has parsed a corpus of 200 Chinese sentences of various types.
An important benefit of a unification-based grammar is that the same grammar can be used both for parsing and generation. Grammar reversibility is a highly desired feature for multi-lingual machine translation application. Following this line, I have successfully applied W‑CPSG to the experiment of bi-directional machine translation between English and Chinese. The machine translation system developed in our Natural Language Lab is based on the shake-and-bake design (Whitelock 1992, 1994). I used the same three grammar modules (W‑CPSG, an English grammar and a bilingual transfer lexicon) and the same corpus for the experiment. As part of machine translation output, W‑CPSG has successfully generated the 200 Chinese sentences. The experimental results meet our design objective and verify the feasibility of our approach.
References
Carpenter, B. & Penn, G. (1994): ALE, The Attribute Logic Engine, User's Guide
Chen, K-J. (1996): "Chinese sentence parsing" Tutorial Notes for International Conference on Chinese Computing ICCC'96, Singapore
Feng, Z-W. (1996): "COLIPS lecture series - Chinese natural language processing", Communications of COLIPS, Vol. 6, No. 1 1996, Singapore
Fillmore, C. J. (1968): "The case for case". Bach and Harms (eds.), Universals in Linguistic Theory. Holt, Reinhart and Winston, pp. 1-88.
Huang, X-M. (1986): "A bidirectional grammar for parsing and generating Chinese". Proceedings of the International Conference on Chinese Computing, Singapore, pp. 46-54
Huang, X-M. (1987): XTRA: The Design and Implementation of A Fully Automatic Machine Translation System, Doctoral dissertation, University of Essex.
Li, L-D. (1986): Xiandai Hanyu Juxing (Sentence Patterns in Contemporary Mandarin), Shangwu Yinshuguan, Beijing
Li, L-D. (1990): Xiandai Hanyu Dongci (Verbs in Contemporary Mandarin), Zhongguo Shehui Kexue Chubanshe, Beijing
Li, W. & P. McFetridge (1995): "Handling Chinese NP predicate in HPSG", Proceedings of PACLING-II, Brisbane, Australia
Li, W. (1996): "Interaction of syntax and semantics in parsing Chinese transitive patterns", Proceedings of International Conference on Chinese Computing (ICCC'96), Singapore
Li, W. (1997a): "Chart parsing Chinese character strings", Proceedings of The Ninth North American Conference on Chinese Linguistics (NACCL-9, to be available), Victoria, Canada
Li, W. (1997b): W‑CPSG: A Lexicalized Chinese Unification Grammar, Doctoral dissertation, Simon Fraser University (on-going)
Lü, S-X. et al. (ed.) (1980): Xiandai Hanyu Babai Ci (800 Words in Contemporary Mandarin), Shangwu Yinshuguan, Beijing
Meng, Z., H-D. Zheng, Q-H. Meng, & W-L. Cai (1987): Dongci Yongfa Cidian (Dictionary of Verb Usages), Shanghai Cishu Chubanshe, Shanghai
Pollard, C. & I. Sag (1987): Information based Syntax and Semantics Vol. 1: Fundamentals. Centre for the Study of Language and Information, Stanford University, CA
Pollard, C. & I. Sag (1994): Head-Driven Phrase Structure Grammar, Centre for the Study of Language and Information, Stanford University, CA
Shieber, S. (1986): An Introduction to Unification-Based Approaches to Grammar. Centre for the Study of Language and Information, Stanford University, CA
Tesnière, L. (1959): Éléments de Syntaxe Structurale, Paris: Klincksieck
Whitelock, Pete (1992): "Shake and bake translation", Proceedings of the 14th International Conference on Computational Linguistics, pp. 784-790, Nantes, France.
Whitelock, Pete (1994). "Shake and bake translation", C.J. Rupp, M.A. Rosner, and R.L. Johnson (eds.), Constraints, Language and Computation, pp. 339-359, London, Academic Press.
Wilks, Y.A. (1975). "A preferential pattern-seeking semantics for natural language interference". Artificial Intelligence, Vol. 6, pp. 53-74.
Wilks, Y.A. (1978). "Making preferences more active". Artificial Intelligence, Vol. 11, pp. 197-223
-------------------------------------
* This project was supported by the Science Council of British Columbia, Canada under G.R.E.A.T. Award (code: 61) and by my industry partner TCC Communications Corporation, British Columbia, Canada. I thank my academic advisors Paul McFetridge and Fred Popowich and my industry advisor John Grayson for their supervision and encouragement. Thanks also go to my colleagues Davide Turcato, James Devlan Nicholson and Olivier Laurens for their help during the implementation of this grammar in our Natural Language Lab. I am also grateful to the editors of the NWLC'97 Proceedings for their comments and corrections.
[1] We leave aside the other components such as discourse, pragmatics, etc. They are an important part of a grammar for a full analysis of language phenomena, but they are beyond what can be addressed in this research.
[2] In formulating W‑CPSG, we use uppercase for feature and lowercase for type; ~ for logical not and / for logical or; number in square brackets for unification.
[Related]
Outline of An HPSG-style Chinese Reversible Grammar ABSTRACT
PhD Thesis: Morpho-syntactic Interface in CPSG (cover page)
Overview of Natural Language Processing
Dr. Wei Li’s English Blog on NLP